==Test== Markdown & Code
Lorem ipsum dolor sit amet, consectetur adipiscing elit. Nullam elementum, ipsum non finibus sodales, tellus sapien pulvinar enim, at sollicitudin felis dui ut lectus. Duis venenatis, lectus a efficitur laoreet, metus eros suscipit mi, nec posuere lorem diam eget eros. Donec porttitor enim eros, et luctus elit gravida at. Praesent venenatis ultrices consequat.
Styling Text
-
To bold text, use
**
or__
. -
To italicize text, use
*
or_
. -
Bold and nested italic text, use
**Bold and _nested italic_**
. -
Deletedtext should use~~
or<del>
, and inserted text should use<ins>
. - Superscript text uses
<sup>
, and subscript text uses<sub>
.
Span Elements
Links
This is an example inline link.
This link has no title attribute.
Refer to a local page on the same server, try About page.
Bloggers get 10 times more traffic from Google than from Yahoo or MSN.
Blockquotes
Blockquotes are very handy in email to emulate reply text. This line is part of the same quote.
Quote break.
This is a very long line that will still be quoted properly when it wraps. Oh boy let’s keep writing to make sure this is long enough to actually wrap for everyone. Oh, you can put Markdown into a blockquote.
Code
Inline code
has back-ticks around
it.
There is a literal backtick (`) here.
A single backtick in a code span: `
A backtick-delimited string in a code span: `foo`
Short code block
Here is an example of AppleScript:
(Every line of codes are indented of the block by at least 4 spaces or 1 tab)
tell application "Foo"
beep
end tell
Another example of Python code:
(Code block is surrounded by triple backticks, and the language python
is specified after the first set of backticks)
s = "Python syntax highlighting"
print s
Long code block
Python
import random
from micrograd.engine import Value
class Module:
def zero_grad(self):
for p in self.parameters():
p.grad = 0
def parameters(self):
return []
class Neuron(Module):
def __init__(self, nin, nonlin=True):
self.w = [Value(random.uniform(-1,1)) for _ in range(nin)]
self.b = Value(0)
self.nonlin = nonlin
def __call__(self, x):
act = sum((wi*xi for wi,xi in zip(self.w, x)), self.b)
return act.relu() if self.nonlin else act
def parameters(self):
return self.w + [self.b]
def __repr__(self):
return f"{'ReLU' if self.nonlin else 'Linear'}Neuron({len(self.w)})"
class Layer(Module):
def __init__(self, nin, nout, **kwargs):
self.neurons = [Neuron(nin, **kwargs) for _ in range(nout)]
def __call__(self, x):
out = [n(x) for n in self.neurons]
return out[0] if len(out) == 1 else out
def parameters(self):
return [p for n in self.neurons for p in n.parameters()]
def __repr__(self):
return f"Layer of [{', '.join(str(n) for n in self.neurons)}]"
class MLP(Module):
def __init__(self, nin, nouts):
sz = [nin] + nouts
self.layers = [Layer(sz[i], sz[i+1], nonlin=i!=len(nouts)-1) for i in range(len(nouts))]
def __call__(self, x):
for layer in self.layers:
x = layer(x)
return x
def parameters(self):
return [p for layer in self.layers for p in layer.parameters()]
def __repr__(self):
return f"MLP of [{', '.join(str(layer) for layer in self.layers)}]"
# This code snippet is from MicroGrad library
C
#define TESTING
#include "train_gpt2.c"
// poor man's tensor checker
int check_tensor(float *a, float *b, int n, const char* label) {
int print_upto = 5;
int ok = 1;
float maxdiff = 0.0f;
float tol = 2e-2f;
printf("%s\n", label);
for (int i = 0; i < n; i++) {
// look at the diffence at position i of these two tensors
float diff = fabsf(a[i] - b[i]);
// keep track of the overall error
ok = ok && (diff <= tol);
if (diff > maxdiff) { maxdiff = diff; }
// for the first few elements of each tensor, pretty print
// the actual numbers, so we can do a visual, qualitative proof/assessment
if (i < print_upto) {
if (diff <= tol) {
if (i < print_upto) { printf("OK "); }
} else {
if (i < print_upto) { printf("NOT OK "); }
}
printf("%f %f\n", a[i], b[i]);
}
}
// print the final result for this tensor
if (ok) {
printf("TENSOR OK, maxdiff = %e\n", maxdiff);
} else {
printf("TENSOR NOT OK, maxdiff = %e\n", maxdiff);
}
return ok;
}
int main(int argc, char *argv[]) {
// build the GPT-2 model from a checkpoint
GPT2 model;
gpt2_build_from_checkpoint(&model, "gpt2_124M.bin");
int C = model.config.channels;
int V = model.config.vocab_size;
int Vp = model.config.padded_vocab_size;
int maxT = model.config.max_seq_len;
int L = model.config.num_layers;
// load additional information that we will use for debugging and error checking
FILE *state_file = fopen("gpt2_124M_debug_state.bin", "rb");
if (state_file == NULL) { printf("Error opening state file\n"); return 1; }
int state_header[256];
freadCheck(state_header, sizeof(int), 256, state_file);
if (state_header[0] != 20240327) { printf("Bad magic state file\n"); return 1; }
if (state_header[1] != 2) {
printf("Bad version in state file\n");
printf("---> HINT: try to re-run `python train_gpt2.py`\n");
return 1;
}
int B = state_header[2]; // batch size, e.g. 4
int T = state_header[3]; // time / sequence length (e.g. 64, up to maxT)
printf("[State]\n");
printf("batch_size: %d\n", B);
printf("seq_len: %d\n", T);
ParameterTensors expected_grads;
float* expected_grads_memory = malloc_and_point_parameters(&expected_grads, model.param_sizes);
// inputs and expected outputs, only used for error checking
int* x = (int*) malloc(B * T * sizeof(int));
int* y = (int*) malloc(B * T * sizeof(int));
float* expected_logits = (float*) malloc(B * T * V * sizeof(float));
float* expected_loss = (float*) malloc(1 * sizeof(float));
// read reference information from Python
freadCheck(x, sizeof(int), B*T, state_file);
freadCheck(y, sizeof(int), B*T, state_file);
freadCheck(expected_logits, sizeof(float), B*T*V, state_file);
freadCheck(expected_loss, sizeof(float), 1, state_file);
freadCheck(expected_grads_memory, sizeof(float), model.num_parameters, state_file);
fcloseCheck(state_file);
// overall OK signal for the test
int allok = 1;
// let's do 10 training iterations, following the pytorch code
float expected_losses[10] = {
5.270007133483887f,
4.059706687927246f,
3.3751230239868164f,
2.8007826805114746f,
2.315382242202759f,
1.8490285873413086f,
1.3946564197540283f,
0.9991465210914612f,
0.6240804195404053f,
0.37651097774505615f
};
for (int step = 0; step < 10; step++) {
struct timespec start, end;
clock_gettime(CLOCK_MONOTONIC, &start);
gpt2_forward(&model, x, y, B, T);
gpt2_zero_grad(&model);
gpt2_backward(&model);
clock_gettime(CLOCK_MONOTONIC, &end);
double time_elapsed_s = (end.tv_sec - start.tv_sec) + (end.tv_nsec - start.tv_nsec) / 1e9;
if (step == 0) {
// error checking at step 0 for reference activations/gradients
// at this point, target should be equal to expected_logits, let's compare
int logits_ok = 1;
float* calculated_logits = model.acts.logits;
float max_diff = 0.0f;
for (int bt = 0; bt < B*T; bt++) {
for (int v = 0; v < V; v++) { // note we only loop to V (ignoring padding)
int i = bt * Vp + v; // linearized index, using Vp
if (i < 10) {
printf("%f, %f\n", expected_logits[i], calculated_logits[i]);
}
float diff = fabsf(expected_logits[bt*V + v] - calculated_logits[i]);
max_diff = fmaxf(max_diff, diff);
if (diff >= 1e-2f) {
printf("MISMATCH AT INDEX %d,%d: ", bt, v);
printf("%f %f\n", expected_logits[bt*V + v], calculated_logits[i]);
logits_ok = 0;
bt = B*T; // to break out of both loops
break;
}
}
}
if(!logits_ok) { printf("NOT "); }
printf("OK (LOGITS), max_diff = %e\n", max_diff);
allok = allok && logits_ok;
// compare the achieved loss
if (fabsf(model.mean_loss - *expected_loss) >= 1e-2) {
printf("LOSS MISMATCH: %f %f\n", model.mean_loss, *expected_loss);
allok = 0;
} else {
printf("LOSS OK: %f %f\n", model.mean_loss, *expected_loss);
}
// finally check all the gradients
int gradoks[16];
ParameterTensors grads = model.grads;
gradoks[0] = check_tensor(grads.wte, expected_grads.wte, V*C, "dwte");
gradoks[1] = check_tensor(grads.wpe, expected_grads.wpe, maxT*C, "dwpe");
gradoks[2] = check_tensor(grads.ln1w, expected_grads.ln1w, L*C, "dln1w");
gradoks[3] = check_tensor(grads.ln1b, expected_grads.ln1b, L*C, "dln1b");
gradoks[4] = check_tensor(grads.qkvw, expected_grads.qkvw, L*3*C*C, "dqkvw");
gradoks[5] = check_tensor(grads.qkvb, expected_grads.qkvb, L*3*C, "dqkvb");
gradoks[6] = check_tensor(grads.attprojw, expected_grads.attprojw, L*C*C, "dattprojw");
gradoks[7] = check_tensor(grads.attprojb, expected_grads.attprojb, L*C, "dattprojb");
gradoks[8] = check_tensor(grads.ln2w, expected_grads.ln2w, L*C, "dln2w");
gradoks[9] = check_tensor(grads.ln2b, expected_grads.ln2b, L*C, "dln2b");
gradoks[10] = check_tensor(grads.fcw, expected_grads.fcw, L*4*C*C, "dfcw");
gradoks[11] = check_tensor(grads.fcb, expected_grads.fcb, L*4*C, "dfcb");
gradoks[12] = check_tensor(grads.fcprojw, expected_grads.fcprojw, L*C*4*C, "dfcprojw");
gradoks[13] = check_tensor(grads.fcprojb, expected_grads.fcprojb, L*C, "dfcprojb");
gradoks[14] = check_tensor(grads.lnfw, expected_grads.lnfw, C, "dlnfw");
gradoks[15] = check_tensor(grads.lnfb, expected_grads.lnfb, C, "dlnfb");
for (int i = 0; i < 16; i++) {
allok = allok && gradoks[i];
}
}
gpt2_update(&model, 1e-4f, 0.9f, 0.999f, 1e-8f, 0.01f, step+1);
// compare the losses
float expected_loss = expected_losses[step];
float actual_loss = model.mean_loss;
int step_loss_ok = fabsf(expected_loss - actual_loss) < 1e-2;
allok = allok && step_loss_ok;
// print the timing information at the end
printf("step %d: loss %f (took %f ms) OK = %d\n", step, model.mean_loss, time_elapsed_s * 1000, step_loss_ok);
}
// final judgement
printf("overall okay: %d\n", allok);
// free everything
free(x);
free(y);
free(expected_logits);
free(expected_loss);
free(expected_grads_memory);
gpt2_free(&model);
return 0;
}
// This code snippet is from https://github.com/karpathy/llm.c
More language examples need to be tested.
Image
Aenean lacinia bibendum nulla sed consectetur. Etiam porta sem malesuada magna mollis euismod. Fusce dapibus, tellus ac cursus commodo, tortor mauris condimentum nibh, ut fermentum massa. The following is a raw <img>
image (Not Recommended):
Cum sociis natoque penatibus et magnis dis parturient montes, nascetur ridiculus mus. The following is a figure with caption:
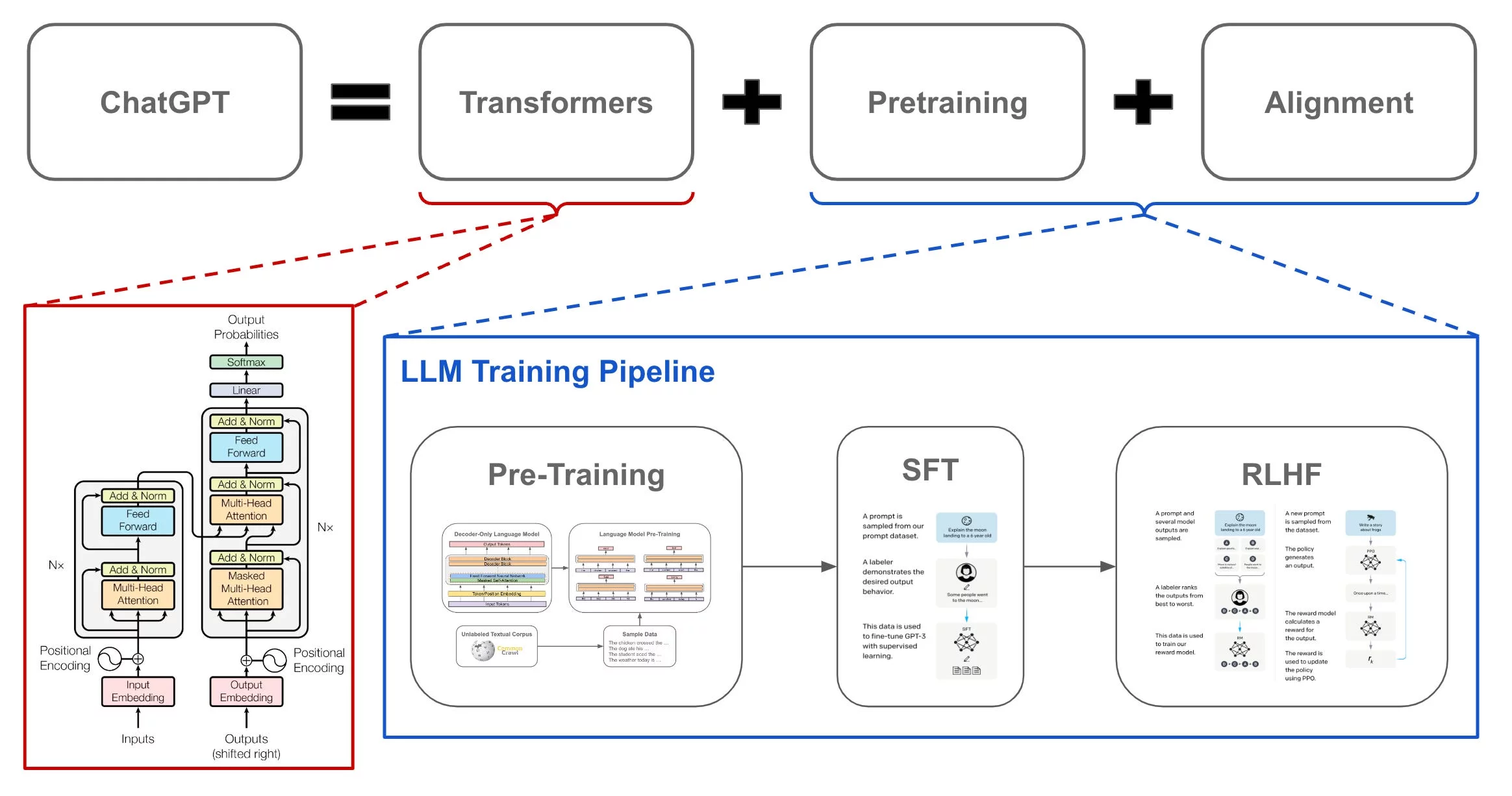
Footnotes
Here is a simple footnote1. With some additional text after it.
This is an example sentence.2
Lists
Normal List
Unordered list:
- Unordered list can use asterisks
- Or minuses
- Or pluses
Ordered list:
- First ordered list item
- Another item
- Unordered sub-list.
- …
- Actual numbers don’t matter, just that it’s a number
- Ordered sub-list
- …
-
And another item.
You can have properly indented paragraphs within list items. Notice the blank line above, and the leading spaces (at least one, but we’ll use three here to also align the raw Markdown).
To have a line break without a paragraph, you will need to use two trailing spaces (There is two unseen spaces after the period in this line).
Note that this line is separate, but within the same paragraph. (This is contrary to the typical GFM line break behaviour, where trailing spaces are not required.)
List with Nested Blocks
-
A list item with a blockquote:
This is a blockquote inside a list item.
-
A list item with a code block:
<code goes here>
To avoid a number being accidentally interpreted as a list item, you can escape the period with a backslash: 1986\. What a great season.
Description List
- Definition list
- Is something people use sometimes.
- Markdown in HTML
- Does *not* work **very** well. Use HTML tags.
Tables
Colons can be used to align columns.
Tables | Are | Cool |
---|---|---|
col 3 is | right-aligned | |
col 2 is | centered | |
zebra stripes | are neat |
The outer pipes are optional, and you don’t need to make the raw Markdown line up prettily. You can also use inline Markdown.
Markdown | Less | Pretty |
---|---|---|
Still | renders |
nicely |
1 | 2 | 3 |
Website is still under development. Expected to be completed by end of August 2024 :D